
Dr. Sophie Naue, Michael Hathorn
Informal settlements are home to one-quarter of the world’s urban population and the number is expected to increase in the coming years. Overall, around 1 billion urban drawlers live in settlements that are often characterized by poor housing conditions and unsecure tenure, located at the urban periphery with limited access to basic services such as clean water, electricity, and sanitation. The improvement of living conditions for these inhabitants remains a pressing global challenge. However, this massive urban growth often leads to outdated records and inaccurate data on deprived areas, which hampers public entities in sustainable planning and responses to resident needs. This raises the question: How can digital technologies help to better understand informal settlements and provide cities with spatial data to support evidence-based planning processes? Considering these challenges, UNITAC Hamburg developed BEAM (Building & Establishment Automated Mapper), a machine learning tool for mapping informal structures using aerial imagery that was piloted in collaboration with the Human Settlement Unit from eThekwini Municipality in South Africa. This project article focuses on the challenges of mapping informal settlements, considering the piloting and development of the BEAM tool in the case of eThekwini.
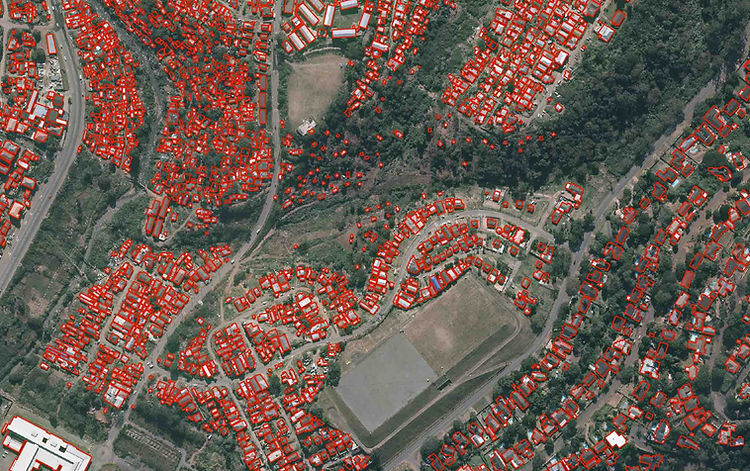
Accurate data is crucial for upgrading informal settlements and enhancing resilience
eThekwini municipality has the largest number of informal settlements in South Africa with over 587 settlements, accommodating over 314,000 households. This accounts for more than a quarter of the entire city’s population.
To address this issue and strengthen community resilience the city has embraced an ambitious informal settlement upgrading programme including basic urban service delivery, housing projects and land management mechanisms. But developing a pipeline of urban upgrading and basic service delivery projects necessitates up-to-date information on the number and location of informal structures as well as environmental constraints that could prevent provision of urban basic services and affordable housing. While eThekwini Municipality is engaged in spatial data collection efforts, data on its deprived areas remains scarce.
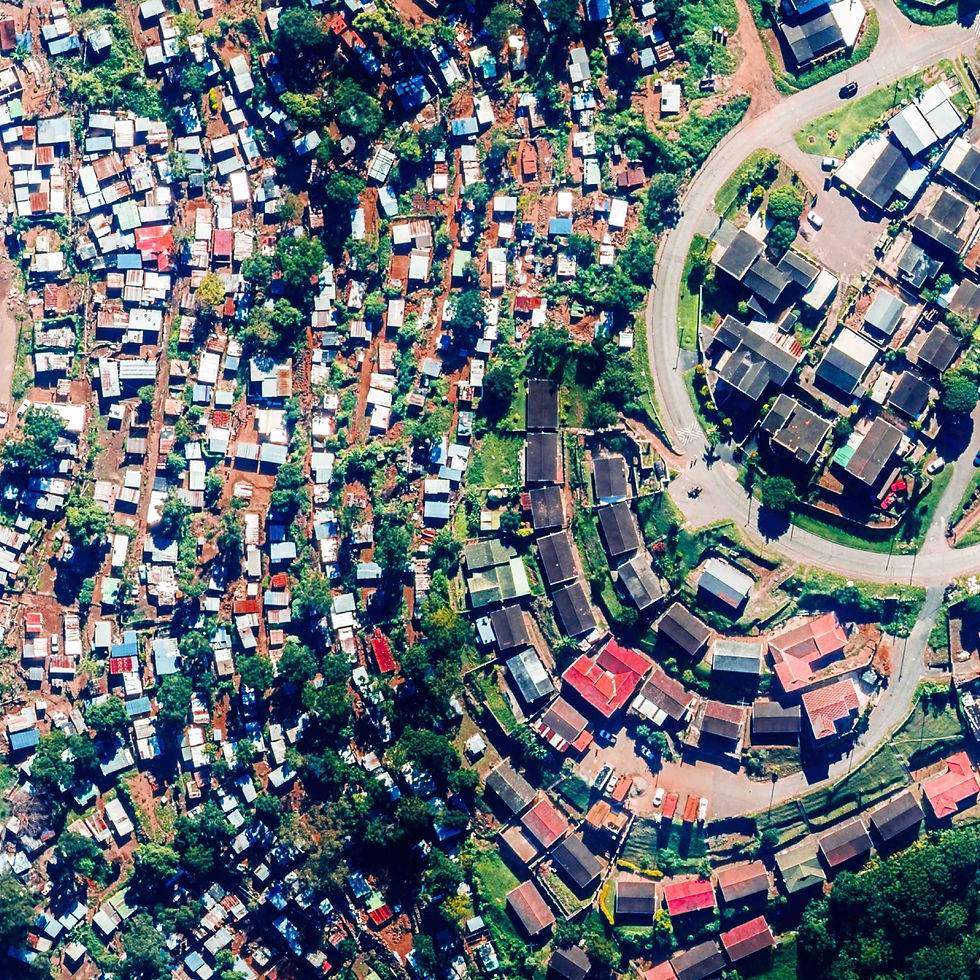
Mapping deprived areas presents a significant challenge due to their unplanned and dynamic nature
Traditionally, spatial data collection relies on survey-based approaches and census data, which continue to be both costly and time-consuming with long gaps between censuses (typically 5 – 10 years) and additional time before the information is available. Given the rapid development of informal settlements, data collected using such methods may already be obsolete when released to the user (Mahabir et al., 2018, p.4).
Considering the above, aerial photography or satellite imagery can provide a detailed view of the physical structure of settlements by facilitating the extraction of building footprints. Although these methods do not capture the intricacies of informal settlements, to say nothing of the characteristics of the people living there, they provide a fast and low-cost picture of the morphology and structure of these areas.
In comparison, traditional mapping remains time-consuming and is a complex process, requiring a nuanced understanding of the political, social, and economic dynamics that shape these neighborhoods. In eThekwini, data has been collected by 15 on-the ground land monitors servicing 587 settlements (eThekwini HSU, 2021). This process cannot keep track of the fast changes in the built environment. The workflow has limited the city’s capacity to respond to residents’ needs.
Another source of information is remotely-sensed images. These include satellite images, lidar data, and 2,500 km2 of annually captured aerial photography, which are manually analyzed and digitalized by the GIS Cooperation of eThekwini (eThekwini HSU, 2021). The size of the areas that need coverage and the rate of change of informal settlements mean that the established processes struggle to capture and maintain data in a robust and timely fashion. These challenges align with UN-Habitat’s recent global study of smart city governance practices (UN-Habitat, 2022), which revealed that less than half of African cities effectively combine manual data with automated data and data from large-scale databases to inform decision making.
Building & Establishment Automated Mapper: Using Machine Learning to map informal settlements
In recent years digital technologies like remote sensing, lidar data, and deep learning have become helpful tools to improve mapping processes of informal settlements. However, developing remote sensing technologies is resource intensive and requires specialized training to operate, which can be a major obstacle for municipalities such as eThekwini. To this end UNITAC Hamburg collaborated with the Human Settlement Unit in eThekwini to develop BEAM (Building & Establishment Automated Mapper). BEAM is a mapping tool for city planners that uses ML to radically accelerate the spatial recognition of informal settlements based on aerial imagery.
The tool was developed as an easy-to-use application that allows the user to quickly detect and visualize urban footprints in a specific area by simply uploading aerial images of a given location. Users have a choice of using one of two trained models (U-Net or HRNet), which offer tradeoffs in speed and performance.

The algorithm is trained to identify and to mark all pixels on an aerial image that the model considers as part of a building. These pixels are converted to georeferenced polygons, which represent building footprints. Those layers can be used to calculate the density of informal settlements in a very easy and quick manner or to identify changes over time, including the expansion, densification, and establishment of new settlements.
Reasons for low identification accuracies of informal settlements are attributed to their rather different morphological characteristics (e.g., higher roof coverage densities, more organic patterns, and small building sizes) compared to formal built-up areas. Due to the heterogeneous characteristics of informal settlement the team had to face various challenges in training the BEAM algorithm. In particular, the model’s performance suffered when being run on building typologies that were not represented in the training set, and on imagery that was not from the same year. To address these issues, a more diverse training dataset was created, and the training pipeline was adapted to make the models more robust on out-of-sample imagery.
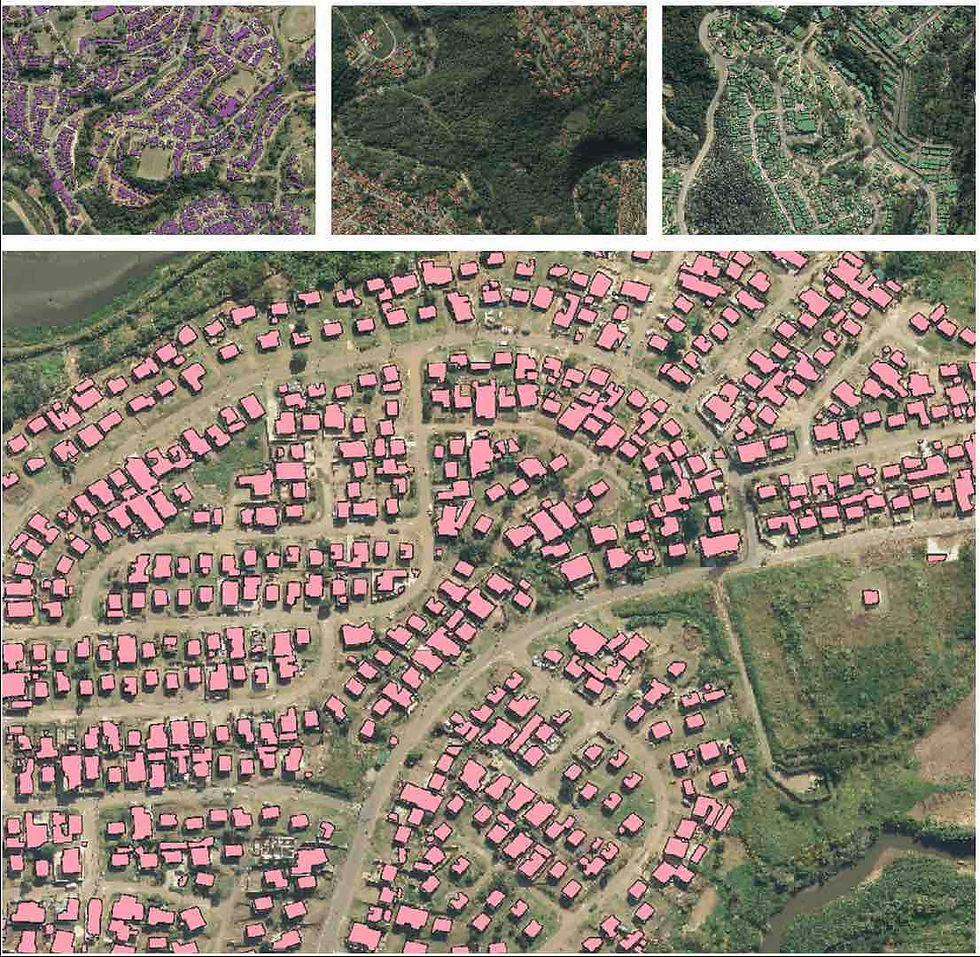
Maintain records of informal settlements’ location, extent, and changes, using BEAM’s outputs for tracking and urban planning
The BEAM tool was co-designed with the Human Settlement Unit, to correspond to their needs and handed over to the city after development. To ensure direct access and advocate local ownership UNITAC also provided a manual, with a step-by-step guidance on how to install and use the tool and conducted serval tech sessions for knowledge and skills transfer, required for the effective use and integration of the tool into the existing workstreams of the municipality of eThekwini. Currently BEAM is being tested by the eThekwini GIS Cop. who has provided feedback and recommendations for improvement.
There are several benefits of using algorithms like BEAM for mapping informal settlements including:
Accuracy: ML algorithms can accurately map informal settlements using aerial or satellite imagery, providing a precise understanding of their location, size, and extent.
Efficiency: Using ML accelerates the mapping process, saving time and resources compared to traditional methods like surveys or manual digitalization, while still delivering reliable data.
Scalability: ML algorithms can be trained to analyze large data sets, useful for monitoring changes over time and identifying areas needing infrastructure development or vulnerable to environmental impacts.
Challenges associated with mapping informal settlements using ML models include:
Data Availability: High-quality data is needed to train ML algorithms effectively. Limited access to aerial or satellite imagery can hinder this process.
Complexity: Informal settlements are diverse, with varying building materials, construction methods, and patterns, making it difficult to develop accurate ML algorithms.
Ethics: ML use raises ethical concerns about privacy, surveillance, and consent.
Maintenance: Continuous updates and training of algorithms are necessary to maintain accuracy and relevance, addressing the critical issues of scale and data access.
Despite the challenges above, today BEAM detects urban footprints on aerial photography achieving an accuracy (Dice coefficient/F1 score) of 91% on an out-of-sample test set. It processes one image in 70 to 90 seconds. With this performance the BEAM tool allows the city to have up-to-date records of the location and extent of its informal settlements as well as to keep track of changes in the built-up area or density over time and leverage the tool output for planning.
Using BEAM eThekwini can reduce the time it takes to map all informal settlements of the city from an estimated 1,320 working days to 72 hours. The tool does the work that otherwise would have taken months and a whole project team. The tool allows the municipality to automate and accelerate mapping processes and achieve more efficient and evidence-based planning processes by understanding the dynamics of Informal settlements.
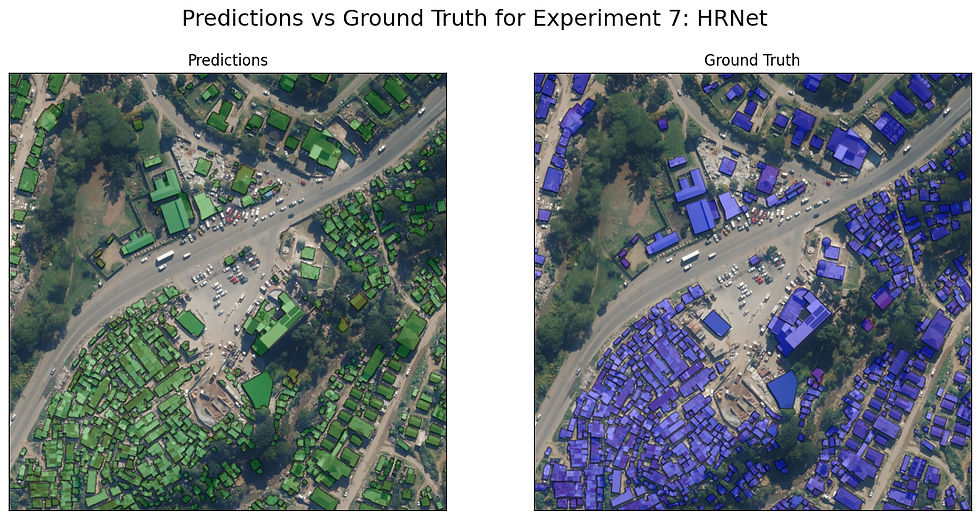
Machine Learning tools enhance urban planning but require ethical use, privacy protection, and community benefits
Mapping deprived areas using ML tools has the potential to provide a more accurate and efficient method of identifying urban growth and density. This can help cities and other policymakers to better understand the dynamics of those areas and inform decision-making processes related to urban planning, housing, and infrastructure development. To manage and understand the massive urban growth, related constraints and opportunities in African cities, digital mapping tools, such as BEAM are critical.
Accurate and up-to-date data is essential to the improvement of public service delivery and enhanced government workflows. Effective urban mapping workflows are vital to streamlining urban service delivery and enabling data-based policies for urban governance and management. Overall, while ML technologies can provide valuable insights of informal settlements there are several challenges and also risks associated with its use. Addressing these will be crucial to ensure that the use of such tools is not only effective but also ethical. Therefore, efforts to map informal settlements must be sensitive to addressing the needs and rights of residents, while also providing decision-makers with information they need to make informed policy decisions. The success of such endeavors relies on ensuring that communities and civil society do benefit from these tools.
To minimize potential risks, it is crucial to establish appropriate data policies that protect privacy and ensure responsible data use. By prioritizing the well-being of residents and implementing the right data policies as well as participatory approaches, we can effectively harness the potential of mapping technologies to create positive change.